Companies, governments, universities, and other organizations have long been calling for innovative solutions for the world’s most pressing problems: climate change, health and well-being, hunger and water scarcity, poverty, and conflicts (Jarvenpaa & Välikangas, 2020). In recent years, rapid technological developments in data storage, computing power, and algorithms have propelled the proliferation of artificial intelligence (AI) applications in business and social landscapes. Due to AI’s ability to identify complex patterns in large volumes of data that are difficult for humans to grasp and subsequently generate accurate predictions, it informs humans with additional information and insights to make decisions. This practical and cost-efficient decision support AI offers can help humans and organizations address humanity’s grand challenges in various ways. For example, AI may help optimize energy consumption, improve renewable energy production, and enhance weather forecasting models to understand better and mitigate climate change’s impacts. In healthcare, AI may improve healthcare outcomes by analyzing large datasets to identify disease patterns, assisting in diagnosis, and personalizing treatment plans. It can also facilitate drug discovery by simulating molecular interactions and predicting potential drug candidates.
For example, Optiml, a Swiss startup, offers an AI-powered solution that creates engineering-grade digital twins of buildings, performs dynamic energy simulations, and optimizes costs and CO₂ emissions (CO₂e). Optiml’s AI algorithms take data about capital expenditure, operating expenditure, energy, CO₂e (Scope 1-3), revenue, and regulatory requirements as inputs. Then, the model’s objective function aims to optimize costs and CO₂e for different investment strategies and renovation plans. Finally, as the output, it provides human decision-makers with the best investment strategies and detailed renovation plans for real estate assets and portfolios, thereby supporting them in achieving “Net Zero Real Estate.” Humans with cognitive limitations struggle to optimize such a large number of input variables at the scale and speed that an AI algorithm can achieve. By addressing the global repercussions of CO2 emissions, such as global warming and climate change, Optiml aims to make a significant impact on sustainability.
In the public domain, the European Centre for Medium-Range Weather Forecasts (ECMWF) has intensified the development of a fully AI-driven forecasting system named the “Artificial Intelligence/Integrated Forecasting System (AIFS).” AIFS is an entirely data-driven weather forecasting model, leveraging an AI model—a graph neural network—trained on historical weather data to predict short to medium-range weather conditions with remarkable accuracy. Recent findings reveal that AIFS surpasses leading physics-based global numerical weather prediction models in several standard performance metrics, including root-mean-square error and the anomaly correlation coefficient, highlighting its superior performance. You can try out AIFS here. By using AI-based systems to forecast severe weather conditions, such solutions help humanity cope with natural disasters.
Despite these reassuring applications in diverse domains, AI is a double-edged sword. Accompanying the great promises and possibilities of AI in addressing the grand challenges is a host of far-reaching unintended consequences related to algorithmic biases leading to social inequalities, privacy, deskilling and job loss, surveillance, accountability, and excessive energy consumption for training and deployment (Berente et al., 2021; Mikalef et al., 2022). In the current landscape, AI dominates discussions in boardrooms and press releases, leaving today’s managers and executives grappling with the challenge of optimizing its capabilities while navigating potential risks and pitfalls.
In this article, we consolidate design principles for AI-based decision support (also referred to as algorithmic decision making) based on our research, aiming to guide executives envisioning prospective AI projects in their organizations to achieve strategic goals or contribute to addressing grand challenges.
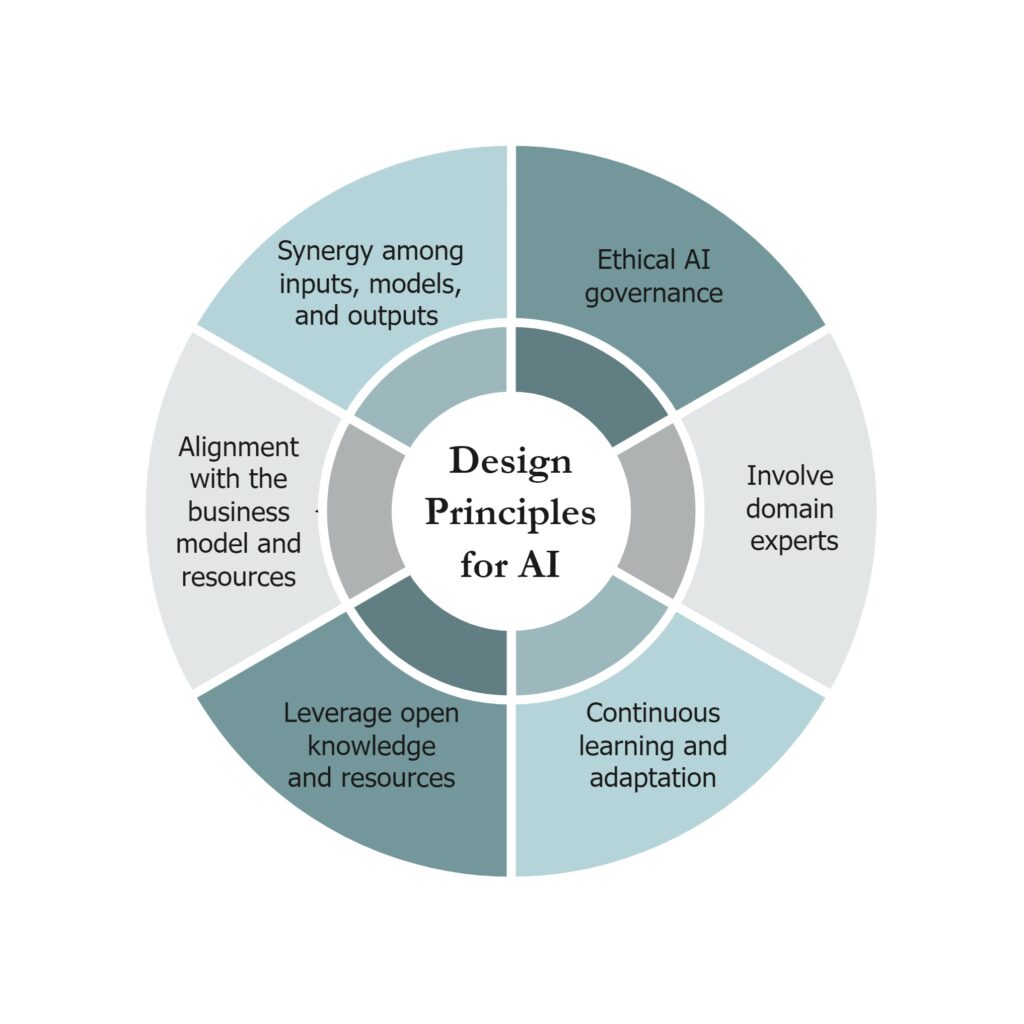
Design Principle 1: Align AI Initiatives with the Business Model and Organizational Resources
To ensure the success of AI initiatives, aligning them with your business model and the organization’s resources and capabilities is essential. Whether the mission is social or corporate, this alignment helps leverage existing resources and ensure that the AI initiatives support and enhance your core organizational objectives.
Design Principle 2: Ensure Synergy Among Inputs, Models, and Outputs
AI initiatives often involve multiple stakeholders, each impacted by the AI project or algorithmic decision-making outcomes. Demonstrating value across these stakeholders necessitates a synergistic approach that integrates diverse inputs (data and domain expertise), optimizes the AI model performance, and ensures the outputs are meaningful and valuable to all parties involved.
Design Principle 3: Implement Ethical AI Governance Frameworks
Establishing robust ethical AI governance frameworks is crucial for gaining and maintaining the trust and engagement of users and stakeholders. These frameworks mitigate employee resistance and reduce algorithmic aversion, fostering a practice of ethical AI usage and ensuring that AI applications are aligned with broader societal values.
Design Principle 4: Involve Domain Experts
Incorporating the knowledge of the domain experts who traditionally contributed to decision-making processes is vital. These experts provide valuable tacit knowledge during the design phase and play a critical role in operational oversight, identifying and correcting AI errors to enhance system reliability and effectiveness.
Design Principle 5: Facilitate Continuous Learning and Adaptation
Given the rapid advancements in AI technology, systems must be designed for continuous learning and adaptation. This includes incorporating new algorithms, hardware, and data sources, as well as adapting to evolving decision-making contexts. Continuous improvement ensures that AI systems remain relevant and effective over time.
Design Principle 6: Leverage Open Knowledge and Resources
AI development can be resource-intensive, and organizations often face financial, talent, and knowledge constraints. Mitigating these constraints involves leveraging community-developed open-source code, utilizing AI/ML libraries for pre-built functionalities, and fostering collaborative partnerships, e.g., industry-academia and outsourcing, to drive innovation and efficiency.
In conclusion, the advent of AI-based decision-making heralds both remarkable opportunities and formidable challenges for modern organizations. Achieving equilibrium amidst the potential benefits and risks necessitates adherence to design principles in real-world AI initiatives. Thus, our actionable design principles, derived from thorough design science research, serve as a guidepost–avant la lettre–for purposeful and ethically responsible AI initiatives in organizations.
Are you interested in reading more about these design principles derived from research conducted with our partner companies? You can explore our recently published empirical research articles, available open-access:
- Herath Pathirannehelage, S., Shrestha, Y. R., & von Krogh, G. (2024). Design principles for artificial intelligence-augmented decision making: An action design research study. European Journal of Information Systems, 1-23. https://doi.org/10.1080/0960085X.2024.2330402
- Herath Pathirannehelage, S., Johannsson, J. G., Shrestha, Y. R., & von Krogh, G. (2023). Artificial intelligence-augmented decision making in supply chain monitoring: An action design research study. In European Conference on Information Systems. https://aisel.aisnet.org/ecis2023_rp/282
References
Berente, N., Gu, B., Recker, J., & Santhanam, R. (2021). Managing artificial intelligence. MIS Quarterly, 45(3), 1433-1450.
Jarvenpaa, S. L., & Välikangas, L. (2020). Advanced technology and end-time in organizations: a doomsday for collaborative creativity?. Academy of Management Perspectives, 34(4), 566-584.
Mikalef, P., Conboy, K., Lundström, J. E., & Popovič, A. (2022). Thinking responsibly about responsible AI and ‘the dark side’ of AI. European Journal of Information Systems, 31(3), 257-268.
Hyperlinks
AI for energy optimization: https://hbr.org/sponsored/2023/09/how-ai-can-help-cut-energy-costs-while-meeting-ambitious-esg-goals
AI for weather forecasting: https://deepmind.google/discover/blog/graphcast-ai-model-for-faster-and-more-accurate-global-weather-forecasting/
AI to improve global health: https://gcgh.grandchallenges.org/challenge/grand-challenges-india-catalyzing-equitable-artificial-intelligence-ai-use-improve-global
Optiml: https://www.optiml.com/en
AIFS: https://www.ecmwf.int/en/newsletter/178/news/aifs-new-ecmwf-forecasting-system
Try AIFS: https://charts.ecmwf.int/?facets=%7B%22Product%20type%22%3A%5B%22Experimental%3A%20AIFS%22%5D%7D
-
Savindu Herath
Savindu is a Sri Lankan research associate and doctoral student at the Chair of Strategic Management and Innovation at ETH Zurich. He is researching the design of AI systems to empower organizations to achieve their objectives with greater efficiency and effectiveness.
View all posts -
Yash Raj Shrestha
Yash is an Assistant Professor at the Department of Information Systems, Faculty of Business and Economics (HEC) at the University of Lausanne and Group Head of Applied AI Lab. His research program aims to identify solutions and frameworks for dealing with organizational and technical hurdles that business organizations face when adopting AI-based systems.
View all posts